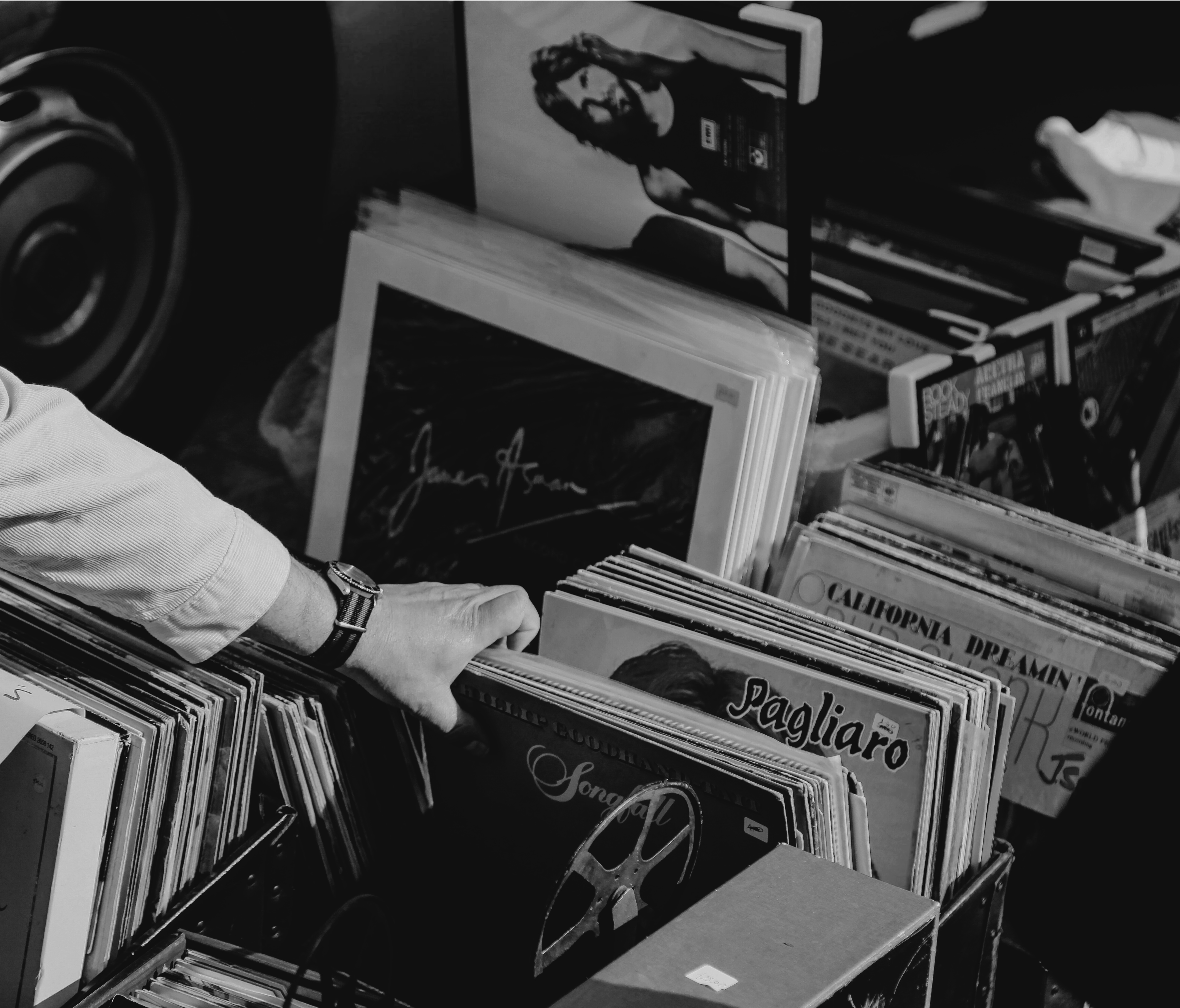
Key takeaways
- Qloo’s Taste AI provides cross-domain recommendations, predicting what customers will love across various categories, from restaurants and entertainment to music and movies.
- With a privacy-first approach, Qloo’s AI operates entirely without processing any Personally Identifiable Information (PII), ensuring full anonymity and compliance with global regulations.
- Qloo’s recommendation models are flexible, allowing businesses to fine-tune suggestions in real-time, adapting to trends and specific audience preferences.
- By combining user interaction signals with rich content metadata, Qloo’s AI delivers highly personalized, nuanced recommendations that resonate deeply with customers.
Your customers’ preferences are more than just data points—they’re a reflection of their tastes, values, and the experiences that shape their lives. Qloo’s Taste AI goes beyond the typical recommendation engine to tap into these deeper connections, predicting not just what your customers might like within a single category, but across the full spectrum of their interests. Whether it’s suggesting the perfect restaurant to match their favorite film or pinpointing the next travel destination based on their taste in music, Qloo’s AI makes cross-domain recommendations that feel personal and intuitive.
Qloo’s Taste AI is like a well-traveled guide who knows every hidden gem in the city—not just where to eat, but the perfect book to read after dinner and the right music to set the mood. This isn’t about following the crowd or sticking to the obvious. Qloo’s AI acts as a cultural connoisseur, discerning the subtle relationships between seemingly unrelated interests to deliver suggestions that your customers will love. You’ll be able to understand not just what people like, but why they like it.
The power of privacy-first AI
Before diving into how it all works, let’s talk about privacy—because it’s the center of Qloo’s approach. Qloo’s Taste AI is designed to operate entirely without processing any Personally Identifiable Information (PII). Everything is identity-free, ensuring that while our AI learns from user behavior, it does so without ever knowing who the users are. This privacy-first approach means businesses can confidently offer personalized experiences without compromising their customers’ anonymity.
The technology behind Qloo’s Taste AI
Qloo’s process begins with a robust data pipeline that ingests vast amounts of information from diverse sources. This data, ranging from customer interactions to content metadata, helps Qloo’s AI build a comprehensive understanding of what people like. Crucially, all this data is anonymized and stripped of any identifiers, ensuring complete privacy.
The AI then processes anonymized user interactions—referred to as “signals.” These signals include everything from a restaurant a customer dined at to the movies they’ve rated highly. These interactions guide the AI in understanding user preferences, but always in a way that keeps individual identities completely out of the equation.
Making sense of the data: entities and embeddings
So how does Qloo’s AI make sense of all this data? Our technology breaks down all these interactions, linking them to “entities.” Think of entities as the individual pieces that make up a person’s preferences—like specific movies, restaurants, or products.
But how does the AI know which entities are similar or related? This is where “embeddings” come into play. While the term might sound technical, embeddings are simply a way for the AI to map out these entities to show their relationships with one another. Imagine a giant map where similar items (like a fast-food restaurant and a popular cola brand) are placed closer together, while very different items (like a rock concert and a yoga clothing brand) are farther apart. This map helps the AI understand what’s related to what, allowing it to make smart recommendations across different domains.
Qloo’s AI uses three types of embeddings:
Taste-based (signal-based) embeddings:
These embeddings are crafted directly from user interactions—like reviews, ratings, or social media engagement. For example, if many users who enjoy Italian restaurants also frequently attend classical music concerts, Taste AI learns to associate these entities. This approach, a form of collaborative filtering, operates on the idea that if User A shares similar tastes with User B in one area (such as restaurants), it’s likely that User A may share other interests with User B (like music preferences). By analyzing patterns across millions of interactions, the AI can predict that a customer who enjoys a particular type of restaurant might also appreciate a specific genre of music or a particular style of fashion. It’s like having a cultural compass that knows where your tastes will take you next. The AI uses models inspired by techniques like Word2Vec and Transformers to understand how these entities relate to each other.
Content-based embeddings:
While taste-based embeddings focus on user interactions, content-based embeddings dig into the metadata of entities. Imagine you’re looking at two movies: one is a historical drama, and the other is a romantic comedy. Even without user interactions, the AI can analyze the genre, director, cast, and even the themes of the movies to understand their relationships. For instance, if both movies share a similar narrative style or a leading actor, they might be placed closer together on the AI’s map. This approach allows Qloo to make recommendations even for new or less-known entities by understanding their intrinsic qualities. Methods such as Latent Dirichlet Allocation (LDA), large language model (LLM) embeddings, and TF-IDF help the AI to interpret these relationships with greater detail and precision.
Hybrid embeddings:
Hybrid embeddings combine the best of both worlds, merging the insights from user interactions with the rich metadata of entities. This dual approach enables the AI to provide even more refined recommendations. For example, if a user enjoys independent films (a signal-based insight) and these films often feature complex, character-driven narratives (a content-based insight), the AI can recommend a novel or a podcast that shares these deep, narrative qualities. This creates a nuanced recommendation that resonates with the user’s broader taste profile, even across different domains.
Delivering cross-domain recommendations with seamless integration
The real magic happens when Qloo’s AI starts generating recommendations. Because it understands the relationships between different entities across multiple domains, it can suggest experiences that cut across categories. For example, if a customer loves Italian food and indie films, Qloo’s AI can recommend a hidden-gem restaurant or nearby independent cinema they might enjoy. These recommendations aren’t just based on what’s popular within a single domain—they’re crafted from a deeper understanding of how an individual’s different interests intersect.
What sets Qloo’s Taste AI apart is its flexibility. The recommendation models are parameterizable, meaning businesses can fine-tune them to generate results that range from niche, unexpected suggestions to more generalized, popular ones. For example, Qloo’s AI can be adjusted to prioritize trending entities that are relevant in recent news or pop culture. Additionally, if a specific audience is provided, the AI can tweak the weight of recommendations to align more closely with that audience’s preferences. This live tuning of content-based model biases allows businesses to adjust their recommendations on the fly, ensuring they align perfectly with their goals.
For businesses, integrating Qloo’s AI with their existing systems is straightforward. Qloo’s Entity Reconciliation Service ensures that the entities in Qloo’s database align with those in the business’s database, facilitating accurate and relevant recommendations. This makes it easy for businesses to leverage Qloo’s powerful AI without disrupting their current operations. For example, if a restaurant in Qloo’s database matches one in your business’s system but with a slightly different name or tag, the Entity Reconciliation Service ensures seamless integration, so the recommendations remain accurate and relevant.
Qloo’s AI is also adaptable. User preferences are constantly evolving, with new restaurants opening, new movies being released, and cultural trends shifting. Qloo’s system is designed to continuously learn and adapt as new data comes in, ensuring that the recommendations remain fresh and relevant. This dynamic adaptability is key for businesses looking to keep pace with their customers’ changing tastes.